Research
My research mainly focuses on QoE of Internet applications, artificial intelligence and machine learning for communication networks, monitoring and analytics of (encrypted) network traffic, proactive QoE-aware and user-centric network management solutions, ML-based configuration of industrial networks, monitoring and orchestration of edge cloud computing services, group-based communications, as well as performance analysis and modeling of communication systems. Below each research topic is explained in more detail.
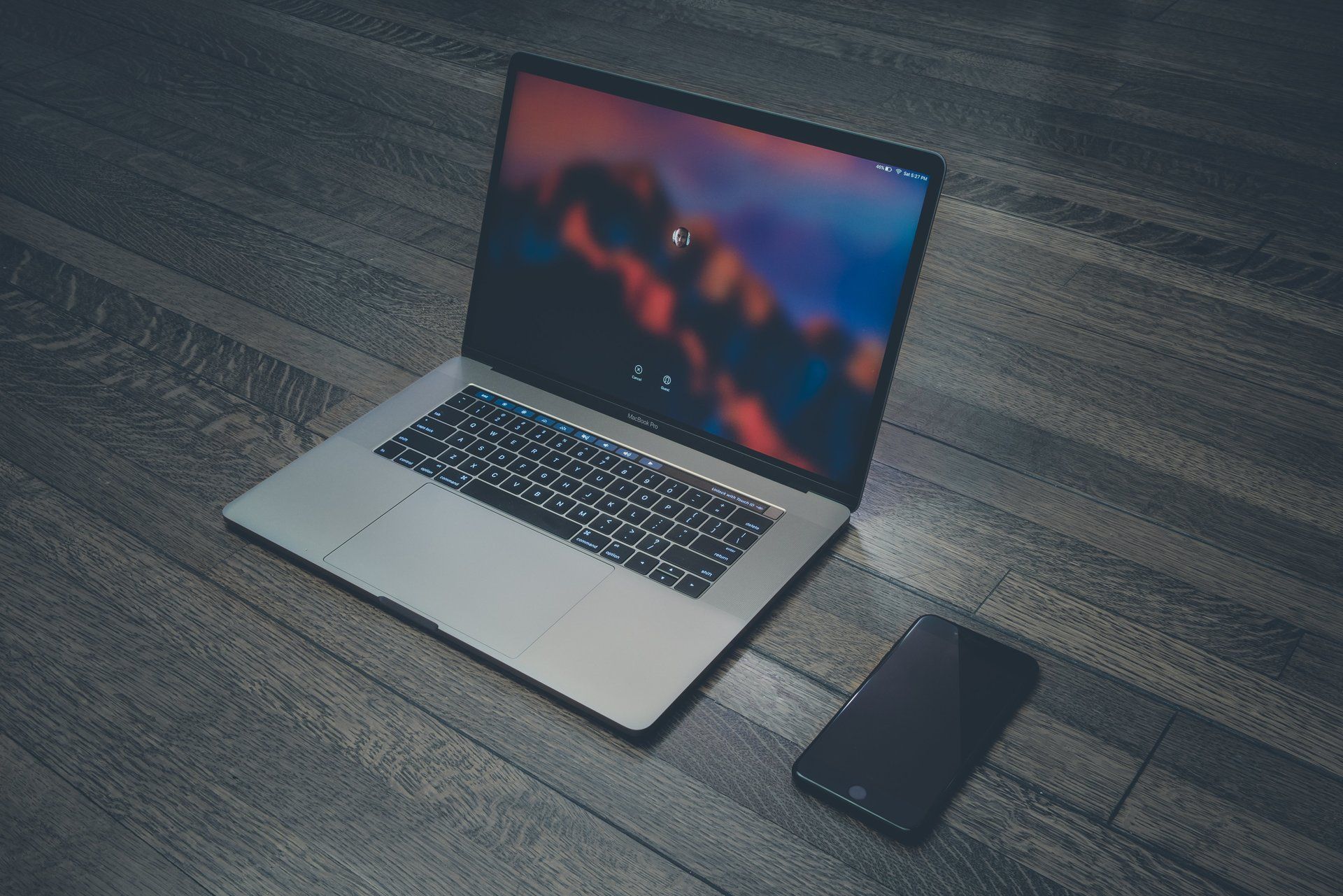
Quality of Experience of Internet applications
In telecommunication networks, the Quality of Service (QoS) is expressed objectively by network parameters like packet loss, delay, or jitter. However, a good QoS does not guarantee that all customers experience the service to be good. Thus, Quality of Experience (QoE) — a concept of subjectively perceived quality — was introduced. QoE is defined as “the degree of delight or annoyance of the user of an application or service. It results from the fulfillment of his or her expectations with respect to the utility and / or enjoyment of the application or service in the light of the user’s personality and current state. [...] In the context of communication services, QoE is influenced by service, content, network, device, application, and context of use” (COST Action Qualinet Whitepaper, 2013). It takes into account how customers perceive the overall value of a service, and thus, relies on subjective criteria. QoE is highly application-dependent and, in order to understand all influence factors, extensive subjective studies have to be conducted.
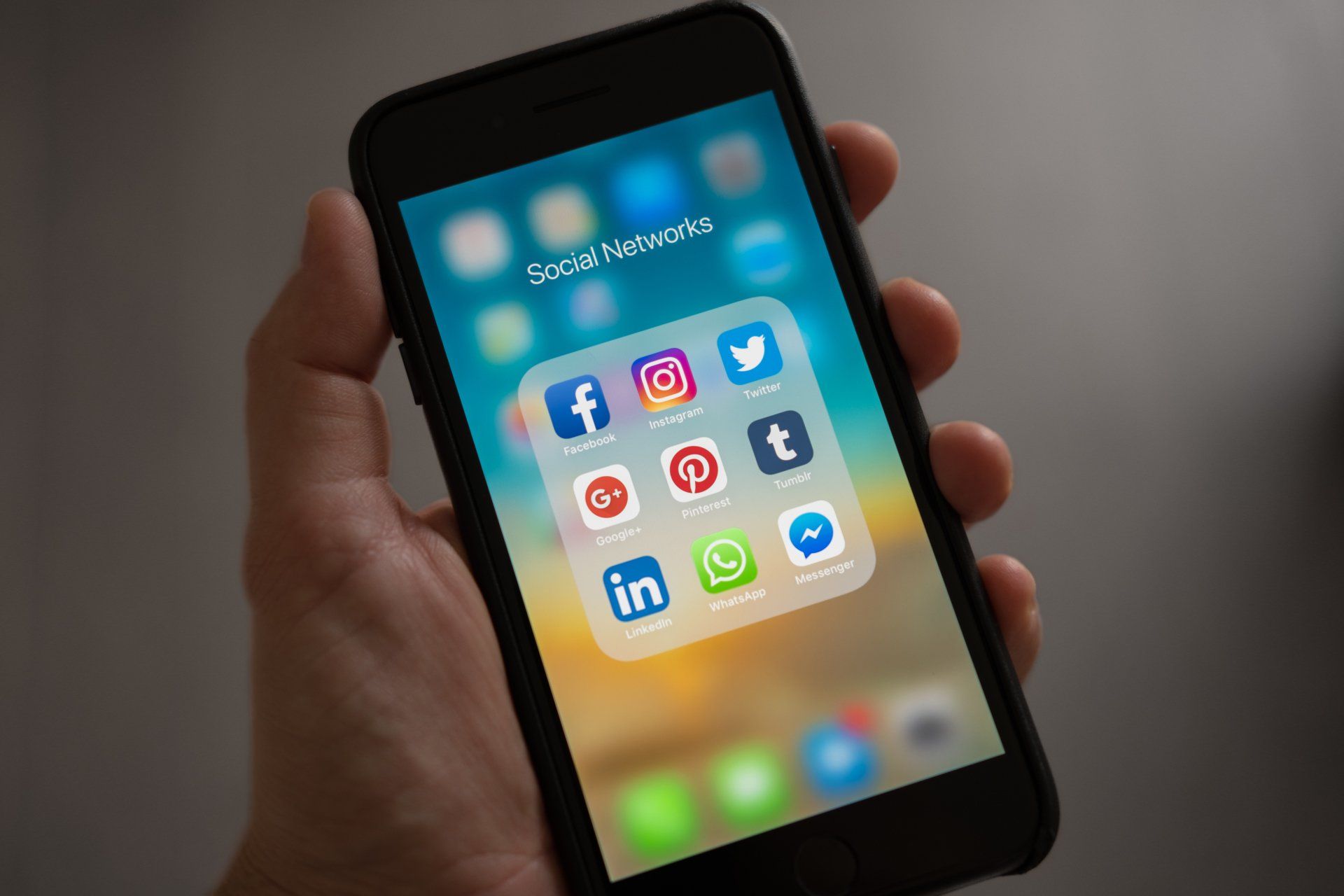
Artificial intelligence and machine learning for communication networks
The advance of handling big data with artificial intelligence and machine learning techniques reaches out from the digitized society as a whole to the networking domain. AI-enabled networks are envisioned, which continuously configure and optimize themselves autonomically (zero-touch) in order to efficiently handle the network traffic, ensure secure operation, and avoid QoE degradations for customers. Towards this proactive network management, in particular, mechanisms are required, which prepare the network in a timely manner for the future network requirements of the users' services. Such proactive traffic management might especially be desired when optimizing the QoE and network security for multiple users with diverse services in heterogeneous networks, e.g., in the context of smart cities, where current and emerging services with different requirements are consumed by a huge amount of concurrent users, and can be delivered via a plethora of mobile and fixed networks.
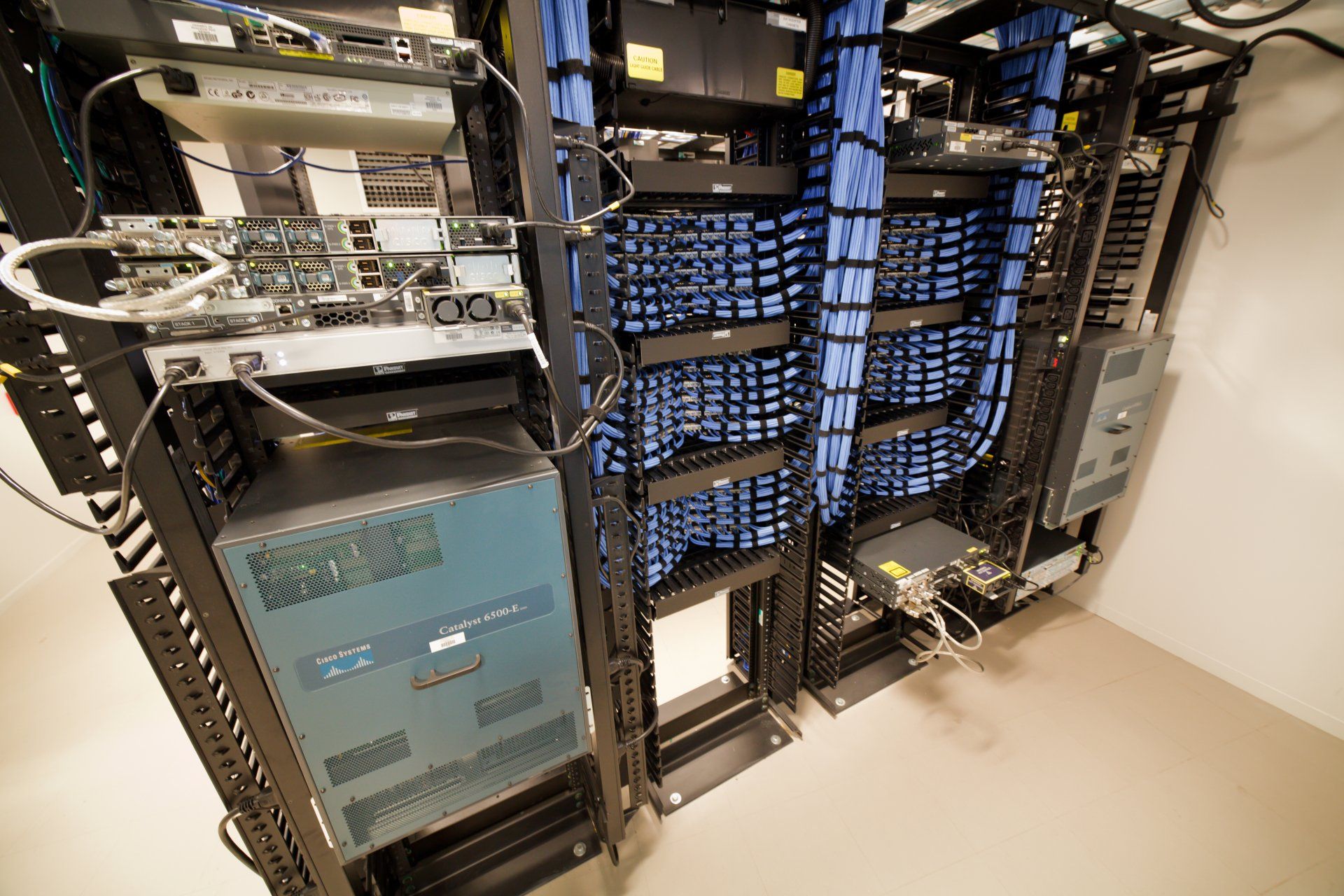
Measurements and analytics of (encrypted) network traffic
To evaluate the current situation in the network, which is the basis of all network management, network operators rely on traffic monitoring to assess the satisfaction of end users and use the monitored information for network management decisions. Typically, they distribute hardware middleboxes or virtual network functions in their networks, which investigate the traffic and extract relevant features, e.g., to estimate the QoE or to assess security threats. However, the recent trend towards end-to-end encryption reduces the visibility of monitoring approaches in the network. Instead, solutions are required, which estimate required information from features that were extracted from the encrypted packet data. Considering the challenge of optimizing QoE, a deep understanding of the underlying network- and application-layer characteristics of the app is required to build accurate (machine-learning-based) QoE estimators for popular apps. Moreover, extendending the QoE concept, user interactions must not be neglected because they can provide valuable information about QoE and network requirements of the application. Thus, monitoring user actions allows to better comprehend the interplay between network and users, which gives possibilities to advance reactive QoE-aware network management towards proactive QoE-aware network management.
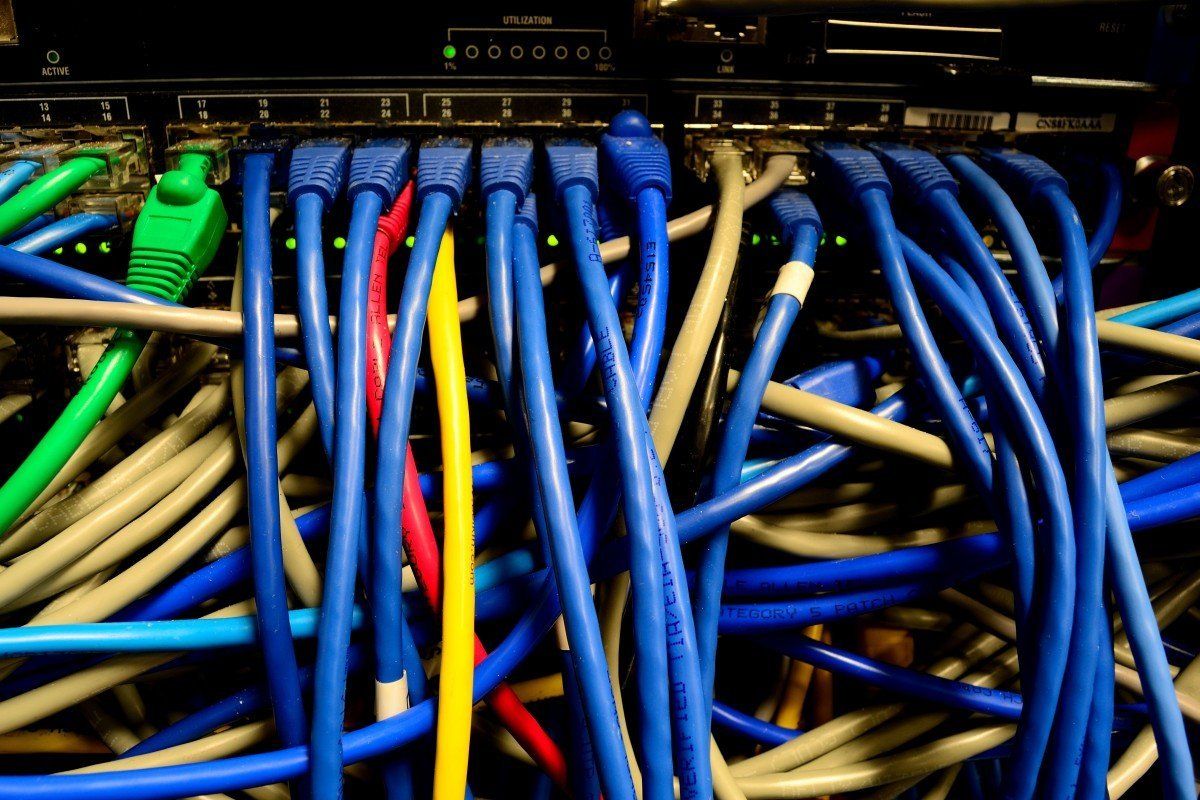
Data-driven proactive user-centric network management solutions
Networks have to be flexibly tailored to the needs of the users, and they need to be operated in an effective, secure, and sustainable way. At the same time, these technologies have to ensure a high subjective Quality of Experience, satisfaction, and security for the users of the Internet services and applications. After the QoE factors of Internet services are known, Internet Service Providers can thus apply network management to improve the perceived service quality. Thereby, especially the concepts of application-aware networks and network-aware applications are investigated and exploited. QoE-aware network management can be extended by additionally considering/involving the end user, his shared resources, as well as social information, e.g., about his preferences, his interests, or his interactions with other users. Instead of only reacting when a QoE degradation has already happened, network management has to become data-driven and proactive in that it learns or even predicts changing network conditions and requirements. This allows to adjust the network configuration in time to completely avoid that the user has to experience QoE degradations and ensure a secure network operation.
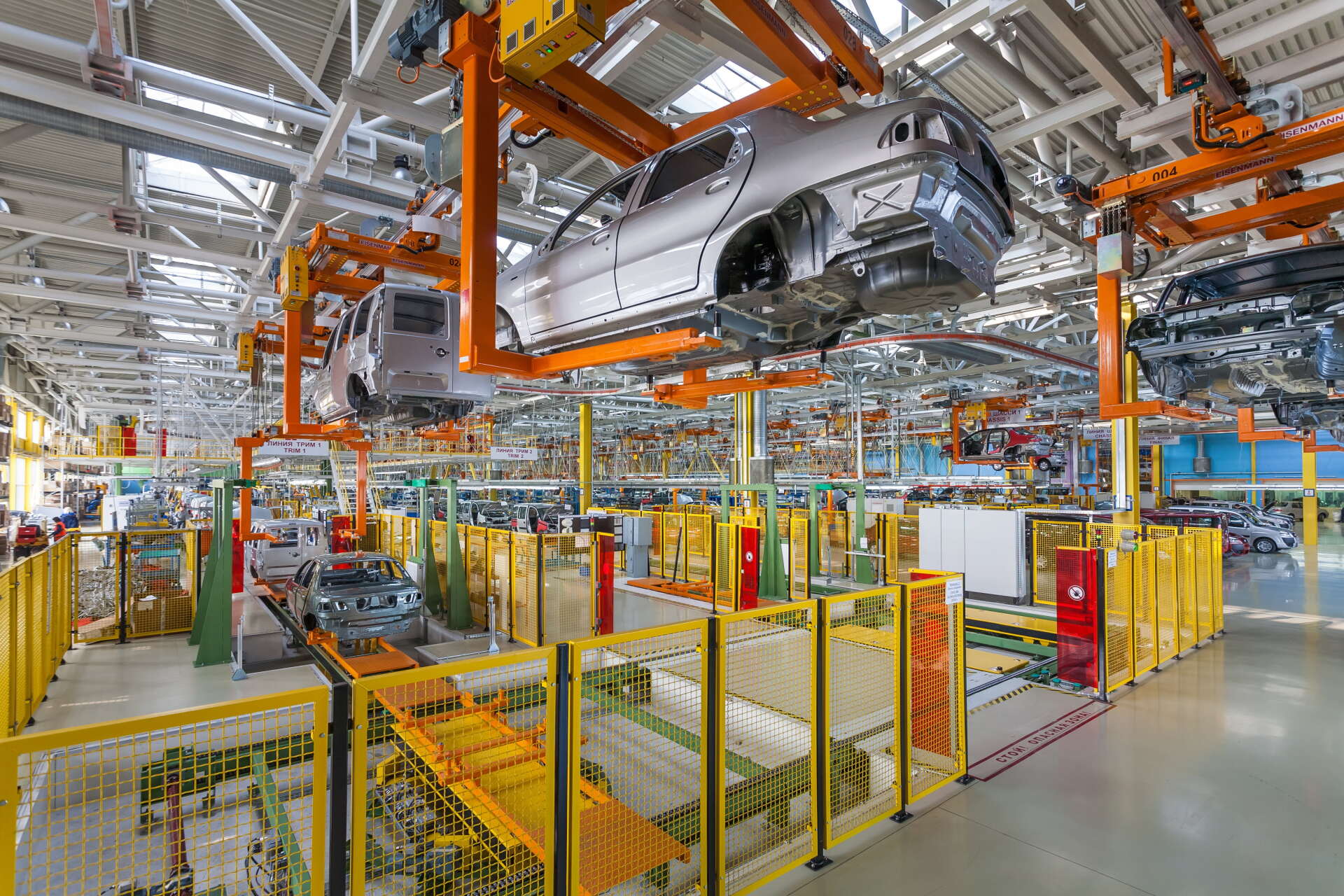
ML-based configuration of industrial networks
Real-time networks in the fields of industrial automation and in-vehicle communication show an increasing demand for both higher data rates as well as higher flexibility. This requires a more dynamic stream allocation considering diverse latency requirements of both critical real-time traffic as well as bursty best-effort traffic. For this, network operators face the challenge to assign delay guarantees to queues such that network utilization can be maximized. Given the huge variety of potential network topologies and stream requirements, classical approaches like exhaustive searches in the parameter space or (integer) linear programs (LP, ILP) are not feasible on short time scales for this type of network configuration optimization problems. Thus, machine learning (ML) could be leveraged to find or approximate optimal solutions more quickly.
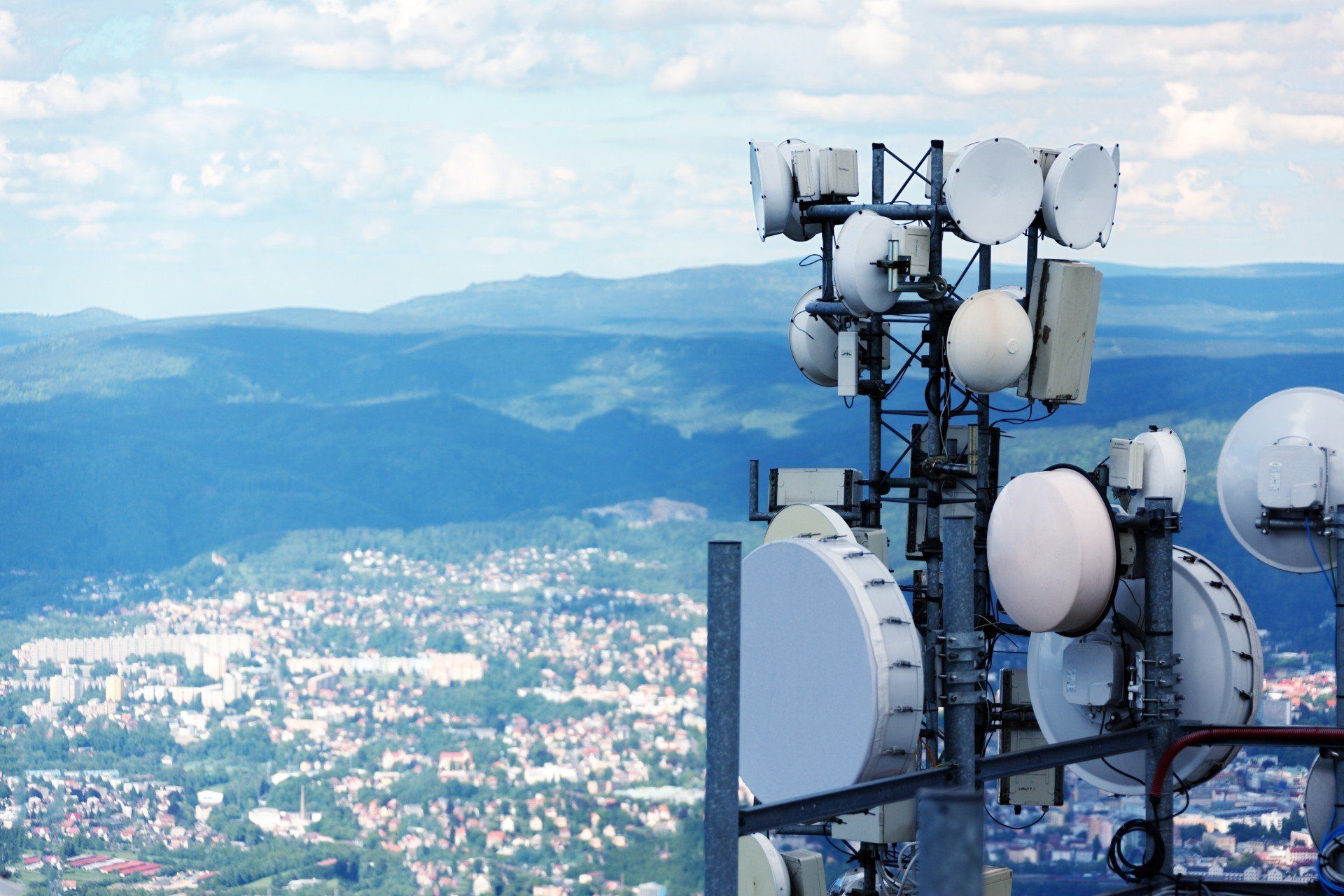
Monitoring, orchestration, and consolidation of edge cloud computing services
Edge computing is a new paradigm that extends cloud computing by additionally utilizing computing resources at the network edge, e.g., servers at mobile base stations or even devices within the homes of end users. By leveraging network function virtualization (NFV) and service function chaining, it allows network operators to reduce capital and operational costs and cope with the increasing demand for flexibly provisioned services. This includes personalized services, which can be instantiated or migrated at the network edge close to end users to support user mobility and achieve a high QoE. The placement of the service apps, i.e., the allocation of VMs to physical servers, of the needed service function chains highly influences the performance of the services and the energy consumption of an edge cloud platform. Therefore, appropriate algorithms for the monitoring, orchestration and consolidation of service function chains have to be designed and evaluated.
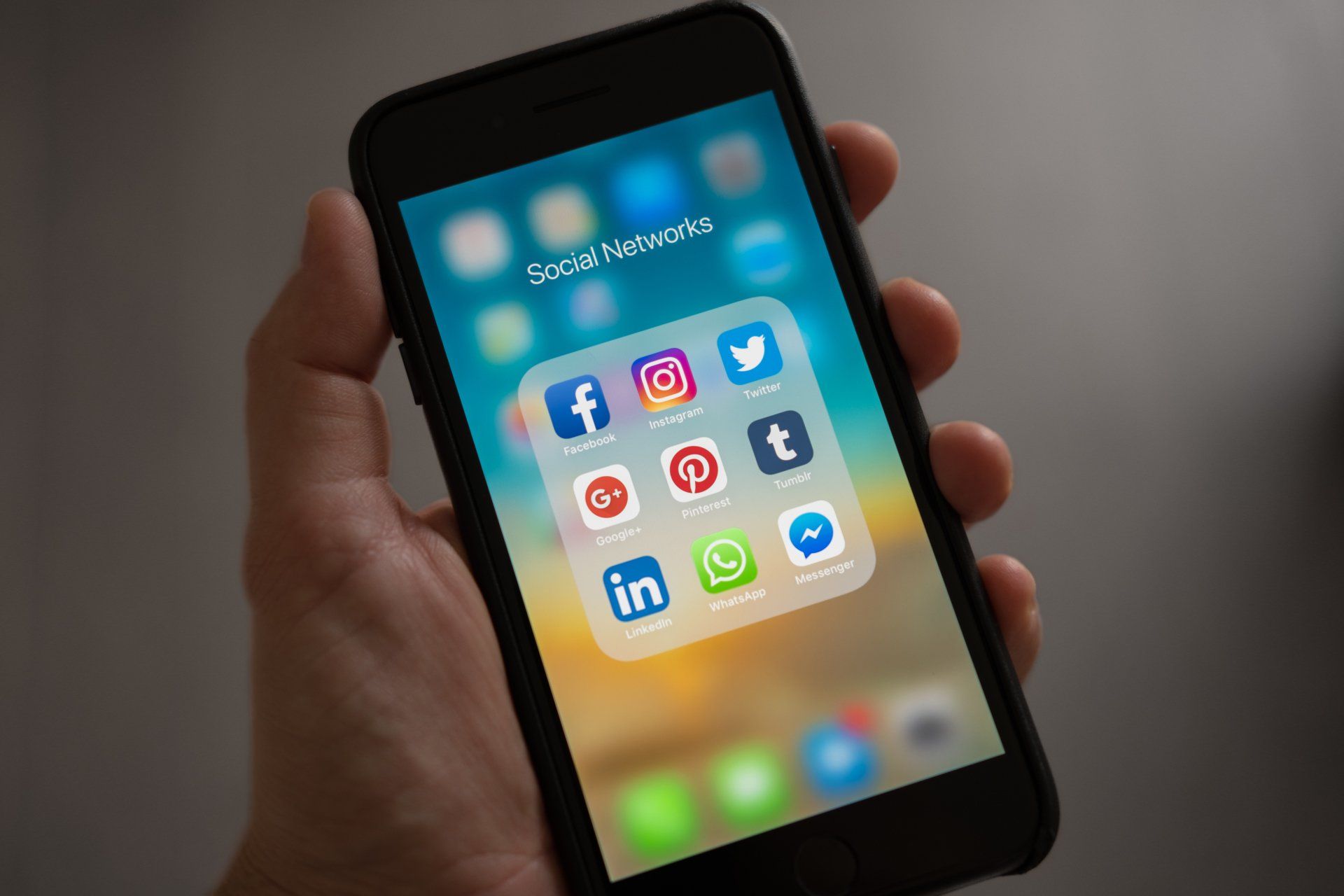
Group-based communication
In recent years, online communication has been supplemented by group communication. Most notably, this evolution is visible for mobile messaging applications, such as WhatsApp. Users are not anymore only communicating one-to-one as it was typical for traditional text-based communication, such as e-mail or SMS. Many social applications also facilitate communicate in groups, which are created spontaneously or which exist over a longer period. Although communication is still asynchronous, due to omnipresent network connectivity and smartphone notifications, the activities of users are often triggered by posted messages in these groups. The increasing usage of such apps, the high data volumes, and the multiplicated transmission of every sent post to all group members put a high load on (mobile) networks. Consequently, the evolving user behavior of group communication might have possible disruptive implications for the future Internet. Models from the past cannot be directly used for group-based communication and need to be adapted to integrate user interactions on a smaller time scale.
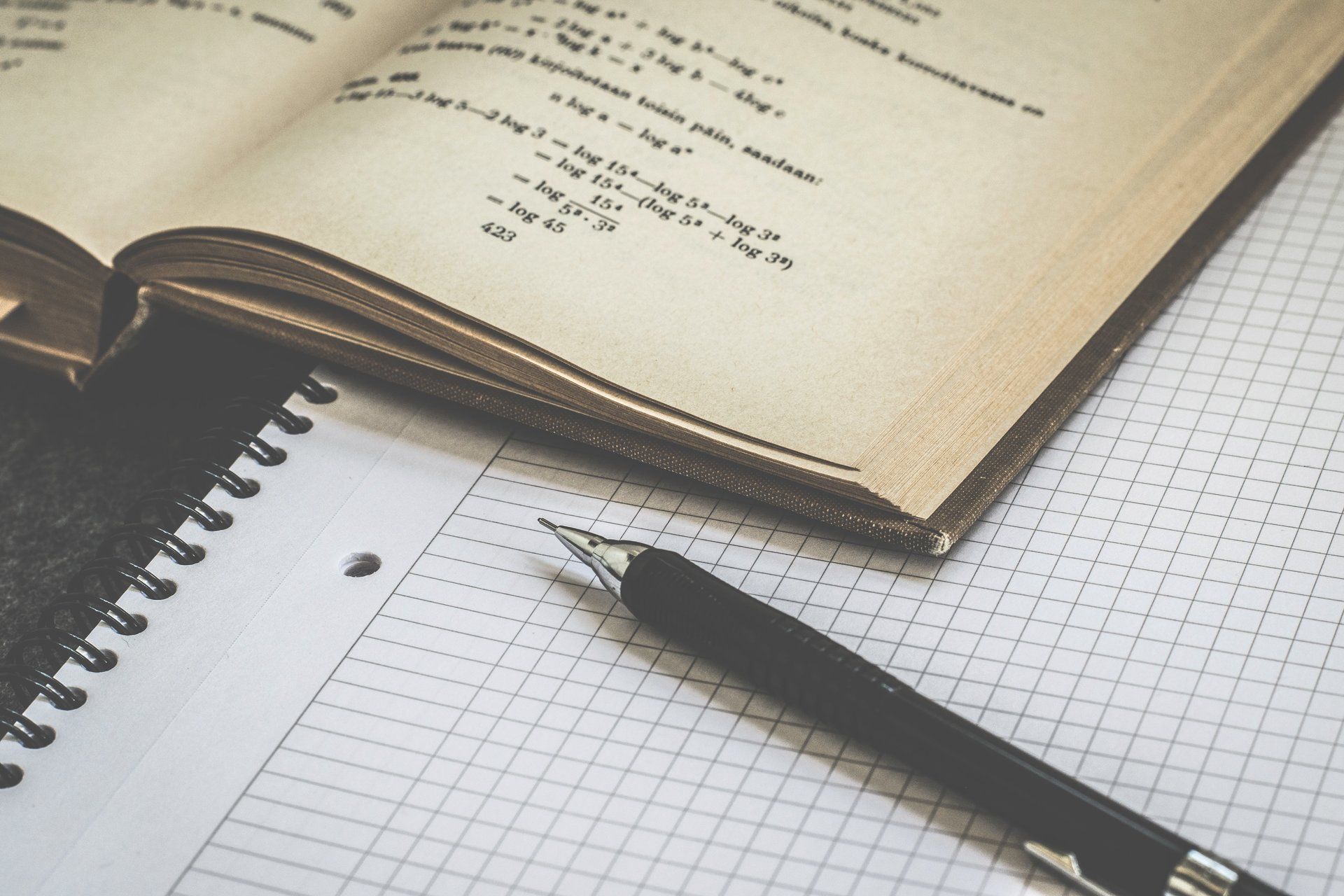
Performance analysis and modeling of communication systems
The performance analysis of communication systems depends on the availability of system characteristics and the desired properties of the resulting models. Analytical methods allow to obtain accurate and scalable performance evaluation results, but are limited to (mathematically tractable) abstract system models. However, they can be used in the planning phase, when a prototype of the real system is not yet available. Simulations can be used to confirm these abstract models, but they can also be used to obtain results for more detailed system models and small problem sizes. Finally, measurements can be applied to evaluate the performance of real systems or networks in detail. The goal of all performance analysis methods is to obtain models, which describe the communication system good enough to detect causes of performance degradations, and to find insights about possible improvements.